Can AI Really Know Your Crops Better Than You Do?
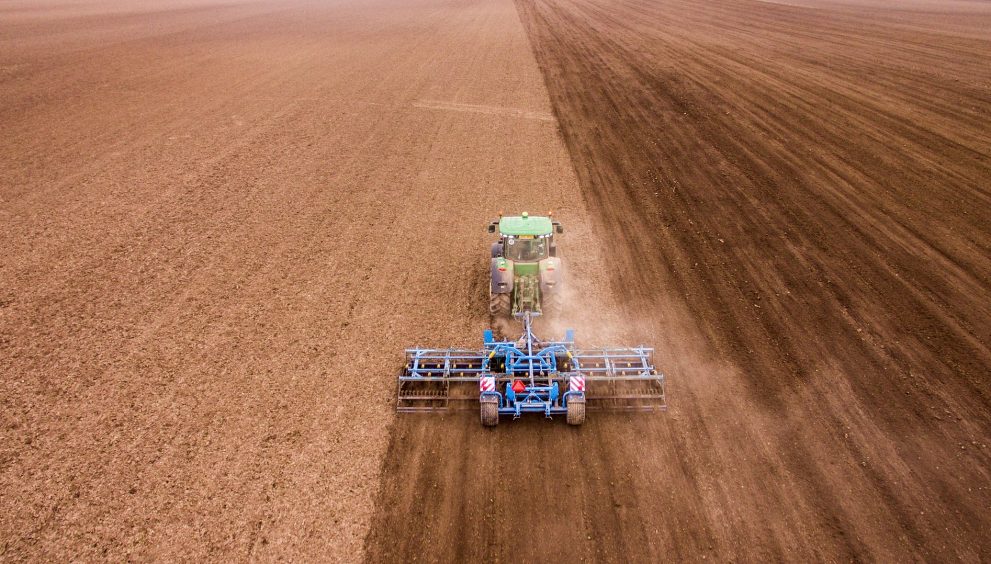
Let’s address the question head-on:
Yes — and not just by a small margin.
In today’s data-driven world, agricultural expertise is no longer limited to what you can observe in the field. Artificial Intelligence (AI) has entered the picture with a level of insight, precision, and foresight that even the most experienced agronomists struggle to match.
This isn’t about replacing expertise. It’s about enhancing it — and, in many cases, outperforming it.
From Experience to Evidence
Traditional crop monitoring depends heavily on field visits, past experience, and manual interpretation of crop conditions. But AI systems don’t rely on memory. They rely on structured, multi-dimensional datasets that span geographies, seasons, and crop cycles.
Platforms like SrajanAI aggregate inputs from:
- High-resolution satellite imagery (optical, multispectral, thermal)
- IoT-based ground sensors (soil moisture, temperature, EC)
- Local weather models and forecasts
- Historical crop performance data
- Remote sensing indices (NDVI, NDRE, SAVI, EVI)
This data isn’t just collected. It’s analyzed, modeled, and converted into real-time, actionable insights that reflect the true state of the crop — not just how it appears to the eye.
What AI Knows That Traditional Monitoring Misses
🔹 Spatial Variability at Scale
AI maps intra-field variability with centimeter-level precision using satellite imagery and drone data. It can detect differences in vegetation health across large farms, something that field visits miss due to time and logistical constraints.
🔹 Pre-Symptomatic Stress Detection
Before visible symptoms emerge, AI algorithms pick up spectral anomalies — indicating nutrient deficiencies, pest infestations, or water stress days or weeks in advance.
🔹 Forecasting Yield with High Confidence
By integrating crop phenology models with historical yield data and ongoing vegetative indices, AI platforms can estimate yield potential early in the season — with improving accuracy as the season progresses.
🔹 Targeted Input Optimization
AI doesn’t suggest blanket inputs. It highlights which zones need nitrogen or irrigation, and at what intensity — drastically reducing waste and maximizing ROI.
🔹 Dynamic Risk Management
With predictive weather overlays and phenological models, AI can alert users to disease onset windows, frost risk, or evapotranspiration spikes — enabling timely decisions.
Why AI Isn’t Just a Tool — It’s a Competitive Advantage
Consider this: no human team can monitor 100,000 hectares daily, adjust for cloud cover, run yield simulations for 5 different climate scenarios, and cross-reference 20 years of data in real time. But AI can — and does.
At SrajanAI, our system fuses satellite signals, ground data, and climatic variables through machine learning pipelines that recalibrate based on in-season anomalies. The result? Not just maps, but decisions:
- Which zones require intervention
- What level of deviation is critical
- What yield is at risk — and why
This level of insight doesn’t just supplement field expertise — it often outperforms it.
Key Advantages of AI-Led Crop Intelligence
Here’s what AI brings to the table:
- Speed: Near-instant analysis of large areas
- Precision: Zone-specific recommendations
- Continuity: Monitoring that doesn’t stop at sunset
- Scalability: Uniform decision support across thousands of hectares
- Objectivity: Pattern recognition free from cognitive bias
Conclusion: Data Doesn’t Lie — It Leads
So, can AI really know your crops better than you do?
Yes — because it sees more, compares more, and never misses a beat.
In a high-stakes, climate-volatile world, relying solely on intuition or traditional scouting isn’t enough. Forward-thinking enterprises are already leaning on AI not just for insights, but for decisive action. This isn’t the future. It’s agriculture’s present — at scale, at speed, and with certainty.