Can Satellite Crop Monitoring Really Be Trusted?
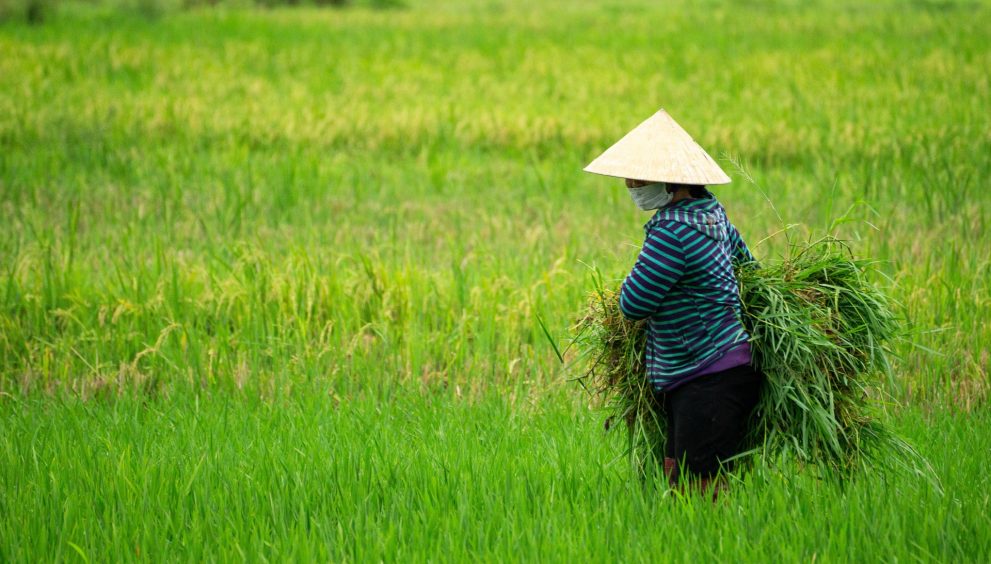
Satellite crop monitoring has emerged as a cornerstone of precision agriculture, offering large-scale, near-real-time insights into vegetation health, crop growth, and environmental stressors. But the question persists: how accurate are these satellite-based observations, and can they reliably guide critical farming decisions?
Understanding the Science Behind Satellite Monitoring
Satellite crop monitoring uses remote sensing technologies to capture data on vegetation indices such as NDVI (Normalized Difference Vegetation Index) and NDRE (Normalized Difference Red Edge Index). These indices measure reflectance values in different bands of the electromagnetic spectrum — primarily red, near-infrared, and red edge wavelengths — to estimate plant vigor, chlorophyll content, and canopy development.
Modern satellites, including Sentinel-2 (ESA) and Landsat-8 (NASA/USGS), offer 10–30 meter resolution and frequent revisit times (every 5–10 days), enabling high-temporal monitoring across vast agricultural regions. Some commercial satellites provide even higher spatial resolution — up to 0.5 meters — but often at the expense of temporal coverage and affordability.
Ground Truthing and Validation
To assess the accuracy of satellite-derived data, researchers conduct ground truthing — the process of correlating satellite observations with physical measurements taken directly from the field. Studies show that NDVI values often have a strong correlation (R² > 0.8) with crop biomass, LAI (Leaf Area Index), and even yield in well-calibrated systems.
In simple terms, ground truthing is the scientific equivalent of verifying a satellite’s assumptions. When a satellite captures data suggesting that a section of a field is healthy — for instance, based on how much light the plants are reflecting — researchers go to that specific location to measure actual conditions on the ground. They might record parameters like leaf area, plant height, or chlorophyll concentration. These measurements are then compared with the satellite’s estimates. If there’s a strong match, it confirms that the satellite data is accurate. This process not only validates current readings but also helps fine-tune the algorithms used, allowing future satellite predictions to become increasingly precise — even without constant on-ground verification.
However, accuracy can vary based on:
- Crop type (e.g., row crops vs. orchards)
- Canopy structure
- Soil background reflectance
- Cloud cover and atmospheric interference
- Sensor calibration and data processing techniques
Limitations and Error Margins
Satellite crop monitoring is not infallible. Limitations include:
- Cloud cover in optical sensors reducing data availability during monsoon or humid seasons.
- Mixed pixels in heterogeneous landscapes leading to spectral noise.
- Data latency affecting time-sensitive decision-making.
Despite these, the error margins in NDVI-based health estimation are typically within 10–15%, which is considered acceptable for macro-level decision-making, particularly in large-scale crop management and seasonal planning.
Enhancing Accuracy with AI and Multi-Source Data
The integration of AI-driven analytics, weather models, and IoT-based ground sensors is significantly improving accuracy. Platforms like SrajanAI employ sensor fusion models that combine satellite imagery with real-time ground data, providing a more calibrated and granular picture of crop health. This hybrid approach helps compensate for satellite limitations, enabling more precise diagnostics and predictions.
By continuously learning from both satellite trends and field-level feedback, these AI models can detect subtle stress indicators that may not be immediately visible through satellite images alone — such as early-stage nutrient deficiencies, pest infestations, or soil moisture imbalances. The system adapts over time, becoming more accurate with each season as it gathers more localized and crop-specific data.
Moreover, multi-source integration allows for dynamic context awareness. For instance, if cloud cover distorts optical satellite readings, the system can shift its reliance toward historical trends, meteorological inputs, and in-situ sensor data. This flexibility ensures a higher degree of reliability, even under variable environmental or atmospheric conditions.
Ultimately, this convergence of technologies doesn’t just improve monitoring accuracy — it enables predictive intelligence at scale, supporting better planning, faster interventions, and more profitable outcomes for large agricultural networks.
Conclusion
While no remote sensing method is perfectly accurate in isolation, satellite crop monitoring provides a scientifically robust and scalable foundation for agricultural decision-making. When combined with machine learning and on-ground calibration, it becomes a powerful tool — not just for observation, but for actionable insight.